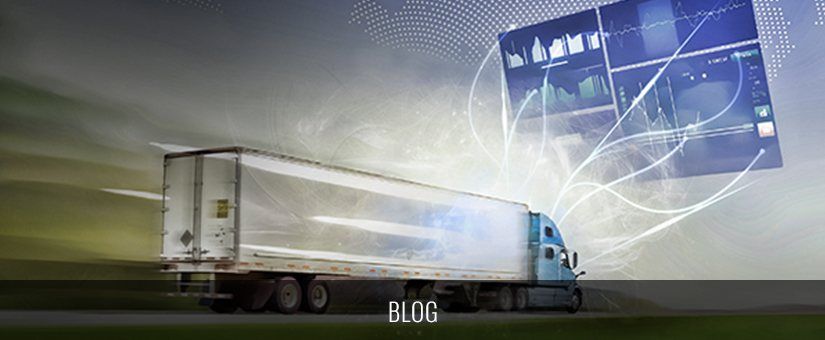
Big Data in Action: The Detection of Unsafe Parking, AKA “Sitting Duck”
By David Wagstaff, Vice President, Analytics, SmartDrive
In my previous blog post, I presented a methodology which could be used to build an analytic solution. In this post I’ll use those steps to define a solution around a significant problem that exists today in the transportation industry: the unsafe parking of vehicles, also known as Sitting Duck.
So, what is Unsafe Parking? Here is a working definition of the unsafe parking problem we are trying to address:
A vehicle that comes to a stop – or has stopped – for an extended period of time, or parked (with ignition on/off) in a lane (stalled) or on the shoulder sitting where parking is not permitted, and for reasons other than traffic jam.
For this post, I’ll be addressing the Sitting portion of the problem statement, i.e., trucks that have parked on the shoulder of a highway.
Why is sitting duck important? In the operation of commercial vehicles, one of the most important operational aspects is safety. Safety means risk elimination at best and risk mitigation at worst. A truck parked on the side of the road represents a significant danger to not only itself and its driver, but traffic on the highway, as well. These situations have continued to increase in recent months due to frequent, and uncertain, COVID-19 closures.
Let’s use the steps from the last blog post to see how they can be applied to this issue.
Steps
Understand and articulate the problem you are trying to solve
The goal is to identify vehicles that are in an unsafe parking situation and remediate the situation as quickly as possible. Remediation could take the form of in-cab alerting to the driver and escalation to supervisors, along with driver coaching to prevent this situation in the future.
Identify data sources and types of data available to answer your business questions
Unsafe parking represents a unique use case in which the detection of the event requires a sequence of events to be detected before the declaration can be made.
For example, the following set of events could be used to identify a Sitting Duck:
- The truck is stopped for at least 5 minutes
- The truck is stopped on, or near (within 25 ft,) a road designated as an interstate
- Prior to the stop, the truck was traveling at least 25 MPH for at least 5 minutes
- The truck is parallel to the direction of travel on the highway
- The truck is not in a designated rest stop
This is just a small subset of the complete list of rules SmartDrive has developed for detection of a Sitting Duck. Having said that, if all the above conditions are met, then it can be inferred that the truck is stopped in an unsafe manner. It should also be apparent that all conditions need to be monitored, and if any are left out of the analysis, the accuracy of the solution is adversely affected by a high false positive/negative rate.
The data list to support these events (which must be available in real-time, i.e., as the events occur):
- The location of the truck (GPS coordinates)
- The speed of the truck, both historical and current
- The bearing of the truck
- The type of road the truck is on
- A list of rest stops, or other areas declared as ‘safe’ for stopping
What do we have?
The SmartDrive system produces real-time telematics data, which is enriched with a vast array of information to determine the risk level at a time and location. This enriched data set covers items #1 – #4
What additional data do we need and can get?
The list of rest stops is currently not available in our data set, so we used two methods to get this data:
- Existing mapping data sources to define rest tops
- Using our large historical telematics database, we applied machine learning techniques to infer locations that appear to be safe areas to park
Define the audience to whom you are communicating your results
There are two groups we are looking to inform (based on our problem statement)
- The drivers
- The drivers’ supervisors and/or fleet safety managers
We desire to have the drivers notified as soon as possible so that they take corrective actions and eliminate the risk. In some cases, there may be legitimate reasons for the truck to be parked; this feedback is important and should be incorporated into the solution.
The supervisors and fleet safety managers are notified if the condition continues to persist after driver notification, i.e., an escalation. This allows additional steps to be performed to remediate the situation.
What additional insights have we learned
As is the case with many programs, we augmented our original problem statement based on information we learned as we built the solution and tested it in the field. A few examples of our learnings:
- We needed to quantify what ‘soon as possible’ was to notify the drivers
A key value we needed to understand was which time period should be used to detect that a vehicle was stopped. - We needed to determine ‘close’ to the highway
This was a tricky problem as there are many ‘safe’ areas adjacent to Interstate roads so the determination of distance to the road is critical to the solution. - We needed to extend our definition of ‘Rest Stops’ to ‘Safe Area’
As it turns out, rest stops are not the only areas that can be safely used as stop areas; refueling stations may have parking areas identified as such. In addition, fleets may have parking areas that happen to be under interstate over-passes. - Allow feed-back from drivers to indicate legitimate reasons for the stop i.e., engine failure. This information should be captured and used to extend the model used to detect unsafe parking.
Summary
Sitting duck situations represent a significant risk to fleets; the ability to identify and correct the situation in real-time provides tremendous benefits.
The process used to identify Sitting Ducks requires real-time data with data enrichment. In addition, the event requires a sequence of events to occur to properly identify the event and eliminate false positives (declaring a Sitting Duck event when it really didn’t happen) while not missing any false negatives (missing a real Sitting Duck event).
By using the steps defined in the prior post, the crafting of the Sitting Duck solution is made more concrete and can be extended as we learn additional information.
Learn more about SmartDrive for Sitting Duck.
Rapid innovation and sophisticated data mining are made possible by the SmartDrive Transportation Intelligence Platform. The analytics team at SmartDrive is continuously solving some of the biggest challenges facing the transportation industry. In my next post, I’ll address another challenge facing the trucking industry – traveling at speeds that are unsafe given the current weather conditions.
- Posted by Melissa.Senoff@smartdrive.net
- On 6 August 2020